
Author: Linda G. Shapiro
Edition: 1
Binding: Paperback
ISBN: 0130307963
Edition: 1
Binding: Paperback
ISBN: 0130307963
Computer Vision
Using a progressive intuitive/mathematical approach, this introduction to computer vision provides necessary theory and examples for practitioners who work in fields where significant information must be extracted automatically from images-- including those interested in multimedia, art and design, geographic information systems, and image databases, in addition to the traditional areas of automation, image science, medical imaging, remote sensing and computer cartography. Download Computer Vision from rapidshare, mediafire, 4shared. The book provides a basic set of fundamental concepts, (representations of image information, extraction of 3D scene information from 2D images, etc.) algorithms for analyzing images, and discusses some of the exciting evolving application areas of computer vision. Th Search and find a lot of engineering books in many category availabe for free download.
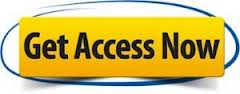
Download Computer Vision
Download Computer Vision engineering books for free. The book provides a basic set of fundamental concepts, (representations of image information, extraction of 3D scene information from 2D images, etc Th
Other engineering books

Computer Vision: Algorithms and Applications (Texts in Computer Science)
Humans perceive the three-dimensional structure of the world with apparent ease. However, despite all of the recent advances in computer vision research, the dream of having a computer interpret an image at the same level as a two-year old remains el


Learning OpenCV: Computer Vision with the OpenCV Library
"This library is useful for practitioners, and is an excellent tool for those entering the field: it is a set of computer vision algorithms that work as advertised."
-William T. Freeman, Computer Science and Artificial Intelligence Laboratory, Ma


Computer Vision: Models, Learning, and Inference
This modern treatment of computer vision focuses on learning and inference in probabilistic models as a unifying theme. It shows how to use training data to learn the relationships between the observed image data and the aspects of the world that we

No comments:
Post a Comment