
Author: Lucian Busoniu
Edition: 1
Binding: Kindle Edition
ISBN: B00918SK90
Edition: 1
Binding: Kindle Edition
ISBN: B00918SK90
Reinforcement Learning and Dynamic Programming Using Function Approximators (Automation and Control Engineering)
From household appliances to applications in robotics, engineered systems involving complex dynamics can only be as effective as the algorithms that control them. Download Reinforcement Learning and Dynamic Programming Using Function Approximators (Automation and Control Engineering) from rapidshare, mediafire, 4shared. While Dynamic Programming (DP) has provided researchers with a way to optimally solve decision and control problems involving complex dynamic systems, its practical value was limited by algorithms that lacked the capacity to scale up to realistic problems. However, in recent years, dramatic developments in Reinforcement Learning (RL), the model-free counterpart of DP, changed our understanding of what is possible. Those developments led to the creation of reliable methods that can be applied even when a mathematical model of the system is unavailable, allowing researchers to solve Search and find a lot of engineering books in many category availabe for free download.
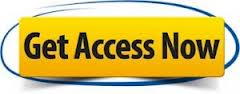
Download Reinforcement Learning and Dynamic Programming Using Function Approximators
Download Reinforcement Learning and Dynamic Programming Using Function Approximators engineering books for free. While Dynamic Programming (DP) has provided researchers with a way to optimally solve decision and control problems involving complex dynamic systems, its practical value was limited by algorithms that lacked the capacity to scale up to realistic problems Those developments led to the creation of reliable methods that can be applied even when a mathematical model of the system is unavailable, allowing researchers to solve
Other engineering books

Reinforcement Learning: State-of-the-Art (Adaptation, Learning, and Optimization)
Reinforcement learning encompasses both a science of adaptive behavior of rational beings in uncertain environments and a computational methodology for finding optimal behaviors for challenging problems in control, optimization and adaptive behavior


Reinforcement Learning: An Introduction (Adaptive Computation and Machine Learning series)
Richard Sutton and Andrew Barto provide a clear and simple account of the key ideas and algorithms of reinforcement learning. Their discussion ranges from the history of the field's intellectual foundations to the most recent developments and applica


Approximate Dynamic Programming: Solving the Curses of Dimensionality (Wiley Series in Probability and Statistics)
Praise for the First Edition"Finally, a book devoted to dynamic programming and written using the language of operations research (OR)! This beautiful book fills a gap in the libraries of OR specialists and practitioners."
-


Prediction, Learning, and Games
This important new text and reference for researchers and students in machine learning, game theory, statistics and information theory offers the first comprehensive treatment of the problem of predicting individual sequences. Unlike standard statist


Reinforcement Learning and Approximate Dynamic Programming for Feedback Control (IEEE Press Series on Computational Intelligence)
Reinforcement learning (RL) and adaptive dynamic programming (ADP) has been one of the most critical research fields in science and engineering for modern complex systems. This book describes the latest RL and ADP techniques for decision and control

No comments:
Post a Comment